Ready to proof -- Clare 03/11/22
KJ proofed 3/11 and sent correction to Clare
Revised 3/14
Ready for author
KJ sent author correction to Clare on 3/18
Revised 3/18 - KJ sent back to author on 3/18
Video: Artem Peretiatko, Creatas Video+/Getty Images Plus, via Getty Images
The Lab of the Future May Be Here Before We Know It
The efficiency of lab research continues to decline, while new innovation challenges emerge
Author — Shriram Ramanathan, Ph.D., Research Director, Lux Research Inc., Boston, MA; Contributor — Cole McCollum
Industries that make heavy use of lab research, such as chemicals and pharmaceuticals, continue to face declining productivity on top of a new set of challenges. These challenges include rising costs with flat or decreased R&D spending, demand for faster development cycles, environmental factors like new regulations, and information overload, where the volume of research data generated grows exponentially every year. These obstacles are putting pressure on companies to do more with less and to adopt digital tools that can improve the efficiency and effectiveness of their lab research.
Momentum for Digital in the Lab Is Growing Steadily
Visions for the lab of the future have evolved over the past few decades, and while individual instruments and processes have benefited from digital tools like automation and analytics, it’s safe to say that lab research has changed far less than many would have predicted. However, with an increasingly broad and powerful digital toolkit that includes artificial intelligence (AI), robotics, and Internet of Things (IoT) sensors, the lab of the future — one that is significantly more automated, efficient, and effective — may be closer to reality.
The Lux Tech Signal — Lux Research's data-driven tool for analyzing innovation interest — shown in Figure 1, adds further evidence to confirm this trend. After an initial wave of hype and activity in the late 1990s, innovation interest in applying digital tools to the lab plateaued for nearly a decade. However, starting around 2013, interest has grown steadily, showing that the space may be in a phase that could lead to a significant impact.
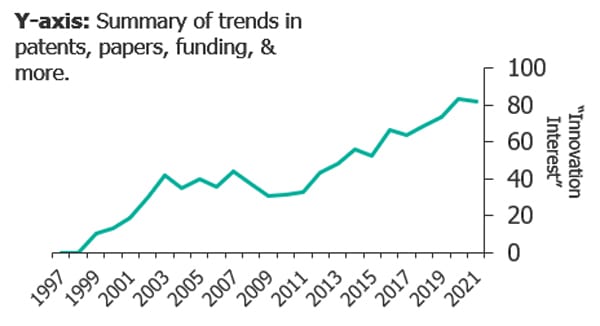
FIGURE 1 ǀ Following a decade-long plateau, innovation interest is growing steadily.
COVID-19 Accelerated the Adoption of Digital for R&D
Despite posing a significant challenge to many R&D teams, the COVID-19 pandemic brought us closer to the lab of the future faster than many would have expected. Due to social distancing measures and regulations, employees who couldn't work physically in a lab turned to digital communication and collaboration tools to remain productive. Working primarily behind a screen also provided an opportunity for employees to explore newer digital technologies, such as informatics tools. Even more radically, some turned to ready-to-go cloud robotic labs like Emerald Cloud Lab and Strateos that allowed them to continue their experiments, even remotely.
Of course, some researchers had to continue going into their labs, but under constrained occupancy limits and other pandemic-related practices, there was still an increased desire for digital tools. For example, some researchers utilized automation to make up for efficiency losses or voice assistants like LabTwin to minimize contacting shared surfaces when collecting data. Overall, the pandemic forced innovation through necessity and provided a catalyst that gave teams an opportunity to rethink their processes from the ground up. While not all these changes will outlive the pandemic, some will, and perhaps just as importantly, the success that many teams saw working through the pandemic will embolden R&D leaders to embrace new processes and digital technologies in the future.
Emerging Digital Tools for the Lab Fall into Three Core Categories
Although there are many digital use-cases and technologies available to enhance lab research, they fall into three broad categories: modeling and informatics, knowledge management, and lab automation. While each category has its own defining features, there is overlap and synergy between categories. For example, lab automation can be used to collect experimental data, which can then be fed into informatics systems to ultimately generate knowledge. Below, we describe these categories in more detail.
Modeling and Informatics
Using modeling and informatics tools like machine learning to accelerate the development and discovery process. Use-case categories include:
- Optimization: Optimizing in silico or designing experiments to optimize existing chemical and/or material structures for a specific structure, properties, performance, etc. Informatics vendors typically focus on optimization, given the strong commercial interest and technical feasibility.
- Discovery: Discovering wholly new chemicals and materials, including synthetic routes. While this is in earlier stages in the materials space, biotech and pharmaceutical companies are very active here.
Knowledge Management
Systematically capturing, analyzing, and distributing knowledge throughout an R&D organization. Use-case categories include:
- Documentation and collaboration: Recording notes and data, and enabling more seamless collaboration. Vendors in this space tend to focus on electronic lab notebooks (ELNs) and laboratory information management systems (LIMS).
- Knowledge search: Searching, analyzing, and extracting insights from internal and external knowledge like research papers and reports. While some companies like Sinequa offer more generic search capabilities, others like PatSnap focus on patent data.
Lab Automation
Automating physical experimentation through robotics as well as data collection and lab management through IoT and connected sensors. Use-case categories include:
- Conducting experiments: Automating experimentation steps. Players in this space include those selling hardware or orchestration software as well as companies using automation for their own research.
- Lab management and data extraction: Monitoring and maintaining lab instruments and conditions, as well as automating the extraction and integration of data from instruments. Vendors in this space include those that sell connected IoT devices as well as software players focused on data extraction.
A Roadmap for Adopting Key Technologies and Use-Cases
By conducting primary interviews with R&D leaders responsible for digital transformation and qualitatively analyzing the current impact and maturity of specific use-cases, we developed an overall roadmap for adopting digital technologies in the lab (Figure 2).
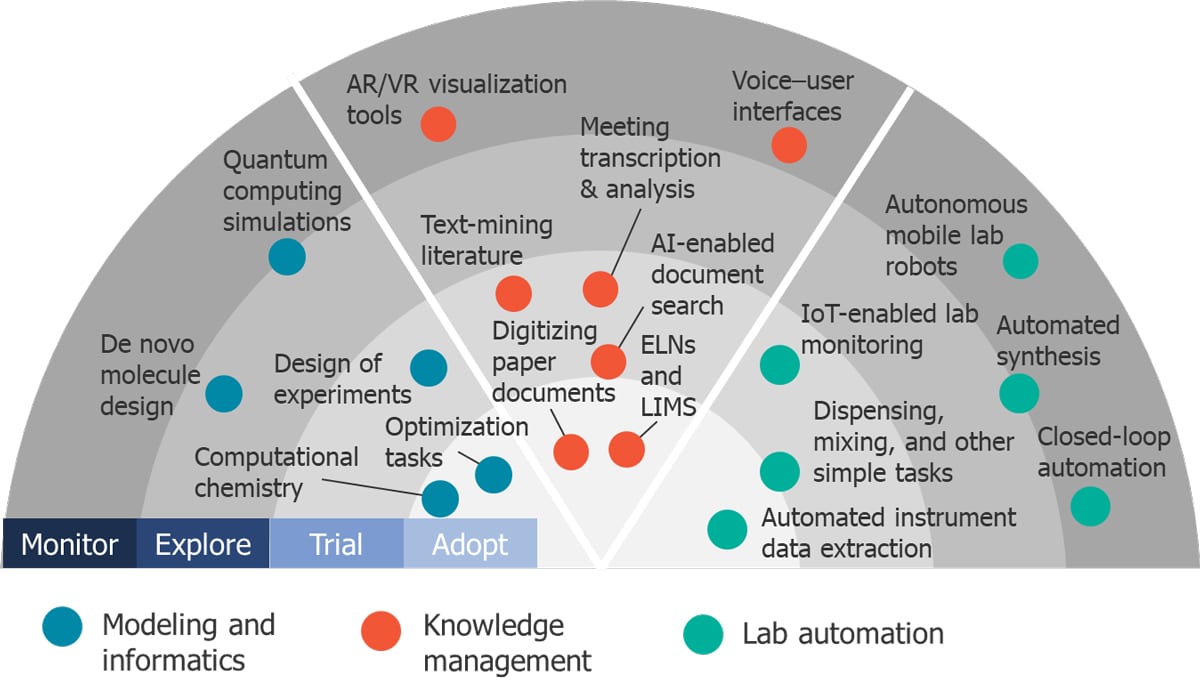
FIGURE 2 ǀ Roadmap for adopting digital technologies in the lab.
AR/VR: Augmented Reality/Virtual Reality
Across the three segments, there are technologies that companies and labs should be adopting today, such as material informatics for property optimization, ELNs, and automated data-extraction tools. We also show much earlier-stage technologies that should be actively monitored or explored, such as quantum computing, voice AI, and closed-loop automation, which will play an important role five to 10 years from now, as well as technologies between these two extremes. Overall, R&D leaders should customize this roadmap to plot a course for their lab of the future.
Digital Will Optimize the Product Development Process and Become a Key Competitive Advantage
Currently, the three segments of modeling and informatics, knowledge management, and lab automation often operate in somewhat separate ecosystems; however, we expect the lines between these areas to grow increasingly blurry over time. As part of this process, we expect to see consolidation in this space, where materials informatics companies may acquire a lab notebook or lab automation company. While companies should avoid lock-in to specific vendors, vendors that create ecosystems of tools that interoperate and share data seamlessly will be well positioned in this space.
As R&D becomes increasingly digitized, we also expect to see more overlap among research, product development, manufacturing, sales (e.g., with digital sales platforms), etc. Instead of predicting just the property of a specific formulation, companies will try to predict the eventual cost to produce the product, where and how it could be manufactured, how long it will take to produce, etc. Once these steps are all digitized, companies can optimize each part of the process and cut down the time it takes to go from research to finished products from years to months or even weeks.
While there is no shortage of near-term challenges in this space, we expect digital capabilities to provide a significant competitive advantage longer term. These data-driven capabilities will compound over time as companies collect more data and use them to understand their customers' needs, strengthening relationships. Eventually, those who effectively invest in and adopt these tools will be able to operate at a speed and level of effectiveness that can't be matched by those who do not. While companies should start by working with vendors to adopt digital tools, they should also set an eye toward bringing these capabilities in-house to build a core competency in this space.
For more information, visit https://www.luxresearchinc.com/.